In the rapidly evolving field of artificial intelligence (AI), Small Language Models (SLMs) have emerged as a compelling alternative to their larger counterparts, offering a blend of efficiency, accessibility, and task-specific performance. This article explores the nature of SLMs, their advantages, applications, and the challenges they present.
Understanding Small Language Models
SLMs are AI models designed to process and generate human-like text but are characterized by a reduced number of parameters compared to Large Language Models (LLMs). Typically, any model with fewer than 30 billion parameters falls into the SLM category. This smaller scale allows for more focused training on domain-specific datasets, leading to models that are both efficient and effective in specialized tasks
Advantages of Small Language Models
Lower Computational Requirements: SLMs demand significantly less processing power, making them suitable for deployment on devices with limited resources, such as smartphones and embedded systems.
Cost-Effectiveness: The reduced need for extensive computational resources translates to lower operational costs, making SLMs an economical choice for organizations.
Faster Training and Deployment: Due to their smaller size, SLMs can be trained and fine-tuned more quickly, accelerating the development cycle and enabling rapid deployment.
Enhanced Privacy and Security: SLMs can be deployed on-premises or within private cloud environments, offering organizations greater control over data security and compliance.
Reduced Latency: Operating locally on devices, SLMs can process data in real-time, providing immediate responses without the delays associated with cloud processing.
Environmental Sustainability: With lower energy consumption, SLMs contribute to a reduced carbon footprint, aligning with sustainable technology practices
Applications of Small Language Models
The versatility of SLMs allows them to be employed across various sectors:
Healthcare: Assisting in diagnostics by analyzing patient data and medical literature to provide insights.
Finance: Automating customer service interactions and analyzing market trends for informed decision-making.
Education: Powering personalized learning platforms that adapt to individual student needs.
Retail: Enhancing customer experiences through chatbots and personalized recommendations.
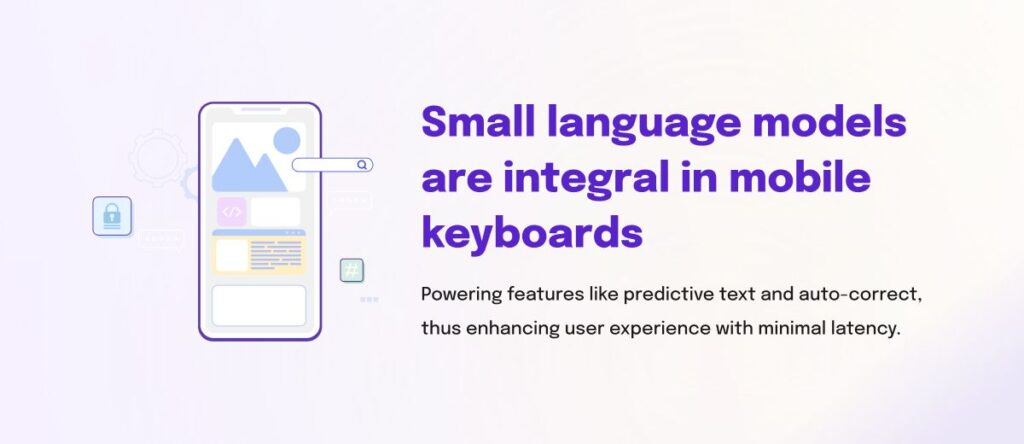
Challenges and Considerations
While SLMs offer numerous benefits, they are not without challenges:
Limited Generalization: Due to their focused training, SLMs may lack the broad knowledge base of LLMs, limiting their applicability to tasks outside their training data.
Potential for Bias: If trained on biased datasets, SLMs can perpetuate and even amplify these biases in their outputs.
Maintenance of Performance: Ensuring that SLMs maintain high performance while operating with fewer parameters requires careful design and continuous evaluation.
Conclusion
Small Language Models represent a significant advancement in making AI more accessible and practical for specialized applications. Their efficiency, cost-effectiveness, and adaptability make them a valuable tool for organizations aiming to leverage AI without the substantial investments associated with larger models. As research progresses, SLMs are poised to play an increasingly prominent role in the AI landscape, offering tailored solutions that meet specific needs while promoting sustainability and privacy
Want to read more interesting blogs like this……Visit https://www.codersbrain.com/blog/
For Career Opportunities visit : https://www.codersbrain.com/careers/.